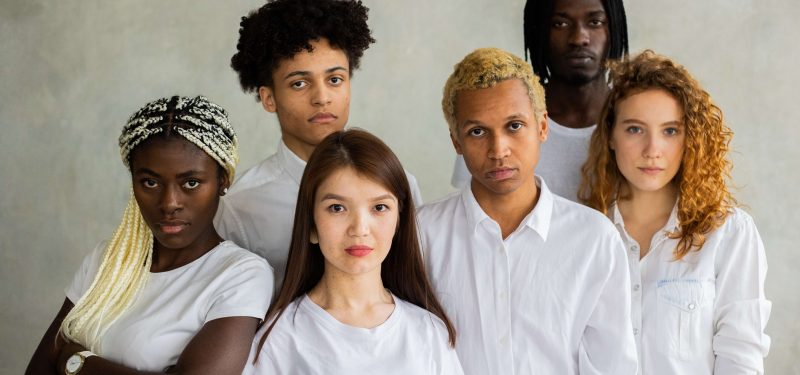
Best Practices for Reducing Bias in AI
Artificial intelligence (AI) is experiencing exponential innovation. ChatGPT, DALL-E, Stable Diffusion, and other AI models have captured popular attention, but they have also raised serious questions about the issue of ethics in machine learning (ML).
AI can make several micro-decisions that impact such real-world macro-decisions as authorization for a bank loan or being accepted as a potential rental applicant. Because the consequences of AI can be far-reaching, its implementers must ensure that it works responsibly. To do that, they must understand bias in AI.
While algorithmic models do not think like humans, humans can easily and even unintentionally introduce preferences (biases) into AI during development and updates. There are three kinds of bias that can affect AI: systemic, human, and computational. By breaking down these different levels, we can address each one effectively and build a robust ethical framework.
Address Systemic Bias by Focusing on Values
As the term indicates, systemic bias is rooted in institutional systems that treat different groups (such as racial categories) differently. This type of bias may be the hardest to address because it is the least obvious. It is also the most foundational of the three because it affects how and when the other two factors surface.
Bias is inherently subjective. It depends on an individual's vantage point. When you try to address systemic issues, the first thing you need to do is find and address the blind spots in your company values. Ask your employees their thoughts about whether they feel the company's values are reflective of everyone at the company and whether those values are being delivered on. Then use that information to evaluate how your AI has been or may be affected. Company values filter from the top down in the organization and can heavily influence the way AI is developed and reviewed, even if it is unintentional.
Another way to address systemic bias is to develop a system of checks and balances. Continually evaluate use cases of your AI over time with several team members who have diverse perspectives and try to scrutinize for new biases, no matter how small. Then bring another team in to confirm and resolve the issues. Set up automated filters that will prevent discrimination or (if applicable) inappropriate language.
Address Human Bias Through Transparency
Human bias occurs when people use their own assumptions and conclusions to fill in information that might be missing. Pinpointing human bias is difficult because AI models operate largely as black boxes; very few people understand how they work.
Work toward transparency in your algorithms. By better understanding why the model returns certain results, you will be able to figure out more easily where bias exists and root it out.
Transparency is a major part of what is called "explainable AI." McKinsey defines this kind of explainability as "the capacity to express why an AI system reached a particular decision, recommendation, or prediction." That kind of transparency, when built into AI, engenders trust because we have a natural inclination to want to understand the reason behind a result—and not just the result itself.
Businesses can work toward this transparency by clarifying what key inputs their AI models use and why. Even if it is a high-level overview, it can help developers pinpoint any correlations to bias that might not have been obvious previously. Businesses can also ensure transparency by undergoing external audits by a neutral third party. Another set of eyes and minds can help stop bias before it ever gets to the user level.
Address Computational Bias with Clean Data
Often, the most obvious and widely discussed issue concerning AI bias is when it is embedded in the training data. This is known as computational bias.
For instance, within conversational voice AI, we see bias issues in even very practical problems that affect end users directly—such as whether an AI bot can understand speakers from diverse backgrounds. Accents and dialects create potential bias scenarios. In a Speechmatics report from last year, nearly 38% of respondents reported that "too many voices are not understood" by voice-recognition technology; another 6.7% reported that "hardly any voices are understood." For a voice AI to be useful, it needs to be trained on data that is representative of all of the people likely to interact with it. Anything less is likely to marginalize some people. But even the best engineers may miss a subtle dialectical distinction, leading to developmental choices that would cause the AI model to misunderstand certain speakers.
Address bias at the computational level by carefully tailoring the AI and training data. Rather than making a general-purpose model, narrow your focus to one interaction that one AI bot can perform. Doing so gives the model less potential for large databased bias. For example, a specific use case for a bot might be collecting customer feedback, while a different use case might provide a claim update—but both functions should not be built together into one bot.
And when you compile training data, make sure that you are removing potential bias as much as possible. That means going back to the different parties involved and engaging end users to uncover what biases they may have experienced.
Also, check for bias by proxy—which can occur when you accidentally introduce bias through secondary characteristics that are correlated to primary characteristics. Harvard Business School professor Ayalet Israeli explained this phenomenon in an interview last year using iPhone cases as an example. She proposed a hypothetical in which women are more likely to buy red iPhone cases than other groups are. In that scenario, Ayalet said, if gender is excluded from an algorithm as a factor but iPhone-case color is included, then the algorithm may nonetheless disproportionately favor (or disfavor) women by virtue of using the color of their iPhone cases as a proxy factor for gender.
AI has the capability to make a significant impact on the way we live our lives and conduct business, but it must be created and maintained responsibly and ethically. By starting from the systemic level, addressing the human element of AI, tailoring AI models, and judiciously vetting training data, you will be much more effective in eliminating bias. Always remember this, however: preventing bias isn't a one-and-done activity; it is something we must consider every day and ingrain in our company cultures.